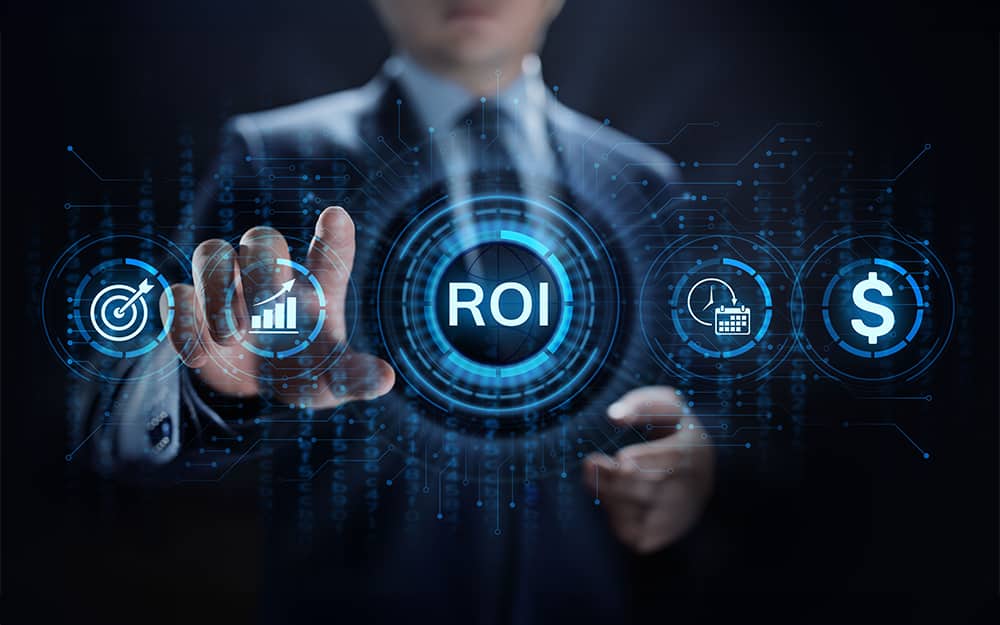
5 Steps to Measure the ROI of the AI Chatbots for Banking (ROAI)
If you think measuring the ROI of an AI bot (ROAI) is simple? Think again!
Can the performance of an AI bot be quantifiable?
While an AI chatbot may not burn out handling quantity, can the quality of CS and CX be measured?
An AI bot is only as intelligent as it is programmed to be. What about the cost of acquiring resources skilled in Machine Learning (ML), Natural Language Processing (NLP), Natural Language Understanding (NLU), Natural Language Engineering (NLE), and Deep Learning?
If you think measuring ROI of AI (ROAI) chatbots in banking is simple? Think again!
Metrics or measurement is the backbone of businesses today. Almost every activity is tracked, measured and its contribution to business mapped.
Almost every team and business meeting involve discussion on metrics. Whether you are an automation expert in a Fintech startup, an owner of a small business or a professional of a big conglomerate, performance metrics that are quantifiable and drive revenue always get the winning vote.
While we have learned to measure regular business activities, doing the same for investments in next-gen technology like artificial intelligence (AI), machine learning (ML) and Big Data poses a great challenge.
Challenges When Measuring Chatbot ROI
The impact and subsequent benefits that AI can deliver for businesses are undeniable. Measuring the ROAI involves certain challenges due to the recency and the wide range of applications of this tech. For example, in case of using AI in marketing the key returns would be revenue driven by bots, increase in conversion rate, Net Promoter Score, etc.
Besides quantitative metrics, there would be some qualitative measurements like internal qualitative feedback from employees and external qualitative feedback from customers. Depending on the industry vertical, metrics might vary. For instance, some of the key performance indicators (KPI) of customer experience (CX) for financial institutions (Fis) and banks is customer loyalty, satisfaction, engagement, retention, and revenue.
Since we cannot cover all verticals and processes, we will focus our efforts to help you measure the ROAI of chatbots in banking related to specific marketing and customer support functions. Let’s get into the number crunching segment of measuring ROI of an AI bot in banking.
Steps to Measure Chatbot ROI in Banking
AI applications may be ‘the next big thing’, but would it enhance your business? What is the purpose of adopting AI technology? What value does it add? No matter how appealing or popular AI applications may be, you need to know if it is relevant and the ROI of your investment.
To break it down into biteable chunks, here are 5 simple steps to help you calculate of ROI of an AI bot in banking:
Step 1 – Identify the queries that can be handled by an AI bot
Examine your knowledge base of queries handled on your live chat. As ‘live person’ chat agents handle queries on a daily basis, asking them to tag common queries could be useful. Some of the examples of common queries on live chats in banking include:
• How to open a bank account?
• What is my account balance?
• How can I pay my bill?
• How can I transfer money?
• How do I apply for a credit card?
Step 2 – Identify the percentage of eligible chats that will be handled by an AI bot
You can identify the common queries that chatbots could handle. This does not mean that chatbots will replace ‘live person’ customer service (CS) agents, it only means that the job market will evolve. As chatbots take care of repetitive tasks, this gives time to CS agents to provide better customer service for complex queries and interactions.
For instance, let us assume that you receive 10,000 chats per month, of which you identify 50% or 5,000 common queries that could be handled by AI chatbots.
Step 3 – Determine the average handling time spent on eligible chats
The time spent on the call in addition to the time spent after the call (known as wrap-up time) would give the average handling time spent on each query. For instance, let’s consider that each of the 5,000 queries:
• The ‘live person’ agent spends 10 minutes on average
• The AI chatbot spends 5 minutes on average (because they are twice as fast)
Step 4 – Calculate the total number of agent hours spent on handling eligible chats
This calculation is as simple as multiplying the number of eligible chats with the average handling time on each chat. In the example, it would be:
• 5,000 chats x 10 minutes / 60 minutes = 833 live agent hours
• 5,000 chats x 5 minutes / 60 minutes = 417 chatbot agent hours
If you want to calculate the precise number of agent hours required, you could use this calculator which applies the Erlang algorithm.
Step 5 – Calculate the annual cost of handling conversations
Your ROAI depends on the efficiency of the chatbot to handle customer conversations or redirect queries from higher to lower cost channels. The true cost of an agent would include costs related to:
• Technology costs – Whether you have cloud-based or premise-based agent deployment, there is a certain hardware and software cost involved in utilizing technology. Consult your IT department to ascertain this cost.
• Utilities costs – This typically includes cost related to internet access, insurance, regulatory compliance, and other utility costs.
• Training costs – Whether you have live person agents or chatbots, a minimal amount of training and programming is required respectively.
Once you have all this information you can derive the true cost of an agent. Assume the hourly cost of a ‘live person’ agent is $15 / hour and the cost of an AI bot is $13 / hour. The approximate annual cost of handling would be the total number of agents hours multiplied by the cost of an agent on an annual basis. In the example, it would be:
• 833 agent hours x $15 x 12 = $150,000 / annum (‘live person’ agent)
• 417 agent hours x $13 x 12 = $ 65,000 / annum (AI bot agent)
Now that you have calculated these figures, how they translate to actionable data and meaningful insights? How do they tell the story of your investment?
The Final Payoff – ROI of AI – How an AI Bot Measures Up
By identifying and determining the average handling time of chats, the agent hours spent on handling chats, the costs of handling conversations, and the cost of an agent (human and bot), you can translate your data into intelligible insights.
The ROI of your AI bot implementation in comparison to deploying a ‘live person’ customer support team would quite simply be the gain from your investment relative to the cost of investment had you not adopted AI technology. In the example, it would be:
The cost-saving from AI bot investment compared to that of ‘live person’ agents ($85,000) – Annual cost of handling conversations by chatbot agents ($65,000) / Annual cost of handling ($65,000) = 31% would be the ROI on AI chatbot implementation. Impressive isn’t it!
Want to dig deeper to calculate the ROI of your AI investments? Mail our industry experts.
ROI on AI – Only a Scratch on the Surface
The calculation of Chatbot ROI on any investment includes multiple parameters and assumptions. While what we have attempted here may be elementary it is as useful. Determining the measurement metrics depends on the area of AI application.
In the case of implementation of AI chatbots in banking, besides cost, an AI bot drives revenue and delivers several other indirect benefits. If programmed accurately, AI chatbots can generate leads and drives sales by cross-selling and upselling. By learning from previous interactions and instances, chatbots have become intelligent in recognizing windows of opportunity to capture a prospect, upsell to an existing customer and make a sale.
We hope this post has got you closer to calculating the ROI of your AI investments. As a further nudge, here is an exclusive sneak peek of the quantifiable benefits measured through the deployment of our AI chatbot for one of our primary clients:
• $852,535 in cost savings by answering 437,000 unique customer queries in 149 days.
• 52% savings in support costs over 6 months
• 28.8% of queries answered by SIA are related to product enquiries and the bank was able to acquire new customers for the various banking products it had to offer.
Know more about how we transformed customer experience (CX) for one of the largest banks in the world, download this Case Study.
Discover the Latest Insights on Interactive Intelligence for Banking Newsletter
Join the newsletter to receive the latest updates in your inbox.